We face new problems every day. Each new problem presents us with unique challenges. Therefore, our approach to solving each business problem should also be unique. However, it is common to observe decision-makers trying to solve every problem with the same tools. It is essential for us to learn the ropes of data analysis for business decision-making.
⚡ Thinking like Sherlock
How does Sherlock crack complicated cases? His deductions are often rooted in the analysis of data.
“Data! Data! Data! I can’t make bricks without clay!” – Sherlock Holmes.
There are some key steps required in data analysis for business decision-making. The first step is the conversion of data into information. The second step requires the conversion of information into insights. Most of the mistakes happen either at the first step. Or at the second step.
Steps for Data Analysis for Business Decision Making
Although different problems may require different kinds of approaches, we can still follow a certain protocol to make the work simpler. Some of the critical steps in data analysis for business decision-making are:
- Formulating the problem.
- Defining the business objectives.
- Developing step by step approach.
- Listing your targets and KPI’s.
- Planning for analysis.
- Converting data into information.
- Extracting insights from information.
Formulating the problem.
People often put cart before the horse. It is quite common to see people jumping right away into extracting information from tables and analyze the data. They start slicing and dicing the data in pursuit of some insights. However, there are several problems with this approach, the most important one is that they have not formulated the problem properly.
Finding the business problems is also an art. Firstly, we need to understand that what is something that we are trying to achieve. This should come as a requirement, either from the customers or from the organization. When these requirements come from the customer side, it essentially means that there are certain pain points for the customers that we’re trying to solve. On the other hand, certain problems arise due to the strategic, operational, or financial issues of a firm.
Defining the business objectives.
Once the problem has been formulated, we also need to formulate the business objectives. Business objectives are the goals that we set for the data analysis process. It is important to understand whose problem we are trying to solve. When we are defining the business objectives, we should also keep in mind the scope of the solution. This scope is the boundary condition for our problems. Often, there maybe three or four different solutions for one particular problem. However, not all solutions are equal.
Therefore, we need to define the business objectives, keeping in mind the feasibility of the solutions. This step also involved the preparation of a summary sheet. This summary sheet should include the benefits and benefactor of the proposed solution. It should also include the expected solutions and scope of the solutions.
Developing step by step approach.
The next step involves preparing a step-by-step approach for data analysis. We have to include the steps that precede and succeed in the data analysis part. Even before we analyze the data, we need to collect it, we need to clean it and we need to prepare it for analysis. Similarly, after the turn Alesis processes over, we need to prepare the data presentation and we need to think about how we’re going to visualize the insights. This planning will help in making the data analysis process more streamlined. In other words, it presents some method to this madness of data analysis.
Listing your targets and KPI’s.
It is also important to list down the target metrics for the data analysis. This may be the set of Key Performance Indicators for your business objectives. For instance, you are analyzing the data to find ways to boost sales in a badly performing geography. In that case, your target KPI may be the number of new leads, sales, or repeat customers. Similarly, you may have any other requirements for your business that get translated into a KPI.
Planning for analysis.
Einstein said, “If I had an hour to solve a problem, I’d spend 55 minutes thinking about the problem and 5 minutes thinking about solutions.” This quote is one of the best guidelines for solving any kind of problem. However, it is priceless for managers to use data analysis for business decision-making. Unless we plan the analysis correctly, we are bound to waste time, develop ad-hoc models and make more errors of judgments. If you want to brush up on your regression skills, head over to our article on guide to multiple regression.
Converting data into information.
Data is useless unless it is converted into information. The process of finding patterns in data is called mining. The reason is that data is like mineral ore, and we have to mine this ore first. Only then we can extract the metal from the ore.
There are various methods and algorithms that we can call as ‘data analysis.’ However, it is quite useful to categorize them into some broad approaches for ease of understanding.
Descriptive | Predictive | Prescriptive | Diagnostic |
Trying to summarize data to make it easier to interpret | Developing statistical models to predict unknown variables | Using data analysis to provide actionable business insights | Attempting to identify problems embedded in the database |
Summarizing & Sorting | Building models | Building Models | Data drilling and knowledge discovery |
Useful to get an overview | Helps in predicting future scenarios | Helpful in formulating solutions | Finding the source of problems |
Extracting insights from information.
Last but not the least, we also need to extract insights from the information. This step requires us to judiciously sift through the output of the analysis. Careful consideration of the model outputs provides us with the required insights. However, it is important to separate useful insights from useless numbers. You may want to use the best method to visualize data. The visualization must answer the need for business analytics.
Common pitfalls to avoid when doing data analysis for business decision making
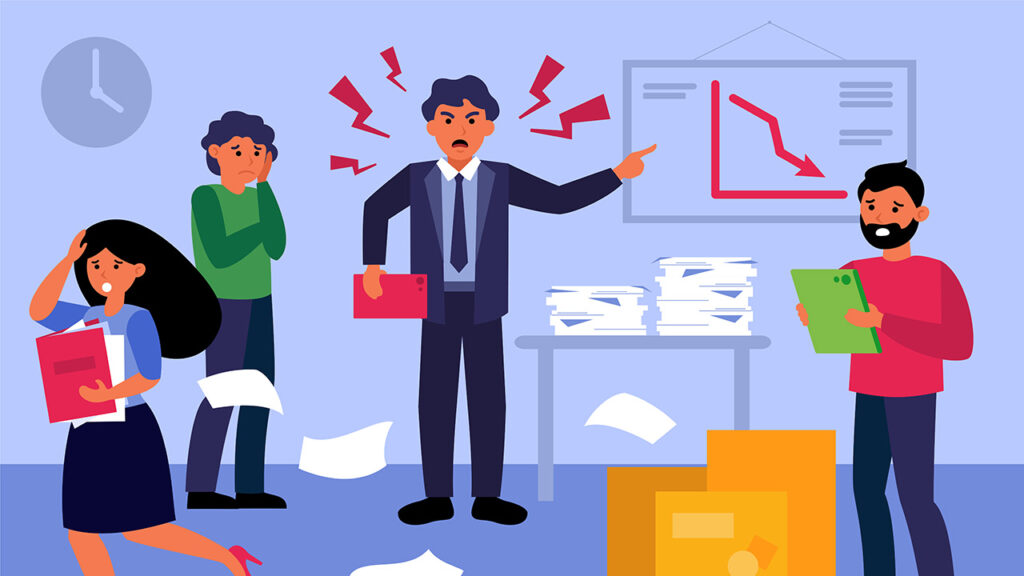
As a good analyst or manager, one must strive to prevent some common pitfalls. Some of these pitfalls are:
- Due deligence – not putting enough effort to understand the business context of data. Any data is meaningless without context
- Mistakes in Model building – these are the methodological errors like using inappropriate model or missing out on some important variable
- Misleading data visualization – it may happen by chance or choice. Best to cross check your output to ensure it does not happen.
- Analysis does not answer the business problem – In this case you may need to back and reassess each step.